画像AIには、”画像認識”、”物体検知”、”セグメンテーション”の三種類があります。
ピクセルレベルで判別する、”セグメンテーション”は詳細に分析できるメリットがあるのですが、数年前までは、実装が複雑で使いこなすのが難しいものでした。
pytorchをベースにした、”Segmentation models”というライブラリを使うと比較的簡単に”セグメンテーション”が実装できますので、そのサンプルコードを解説します。
初心者向けに、難しい用語はなるべく使わずに説明します。
はじめに
”Segmentation models”のページはこちらです。
今回解説サンプルコードはこちらになります。
pytorchではなく、tensorflwo kerasでのセグメンテーション実装はこちらです
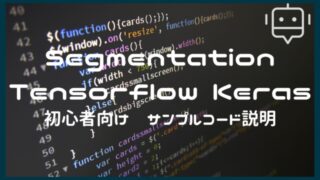
サンプルコード説明
サンプルコード解説です。こちらは、”binary segmentation”です。
犬・猫 または それ以外 の 二分類のコードになります。シンプルなので、初めに試してみるにはぴったりです。
主に4構成になっています。
- データセットとデータローダ(データを取り出すところ)
- LightningModuleの設置(学習に便利なモジュールを使ってい設定します)
- IoUという指標でセグメンテーションの精度を計測するところ
- 結果の可視化(実際にセグメンテーションしてみた結果ですね)
ライブラリ等の準備
!pip install segmentation-models-pytorch
!pip install pytorch-lightning==1.5.4
ライブラリのインストール。colba上での実行例です。コマンドラインでの実行は ”!” を取ってください。
- 1行目:Segmentation modelsをインポートします。
- 2行目:pytorch lightningをインポートします。
import os
import torch
import matplotlib.pyplot as plt
import pytorch_lightning as pl
import segmentation_models_pytorch as smp
from pprint import pprint
from torch.utils.data import DataLoader
ライブラリのインポートです
- 1行目:ファイル操作のos
- 2行目:pytorch
- 3行目:グラフ描画のmatplot
- 4行目:とても便利なpytorch lightning
- 5行目:今回主役のSegmentation models
- 8行目:データの読み込みに便利なデータローダ
データセット
from segmentation_models_pytorch.datasets import SimpleOxfordPetDataset
“Segmentation models”に用意されている、”シンプル オックスフォード ペット データセット”というものを使います。
# download data
root = "."
SimpleOxfordPetDataset.download(root)
データセットをダウンロードします(rootは保存先フォルダのパスです)
# init train, val, test sets
train_dataset = SimpleOxfordPetDataset(root, "train")
valid_dataset = SimpleOxfordPetDataset(root, "valid")
test_dataset = SimpleOxfordPetDataset(root, "test")
# It is a good practice to check datasets don`t intersects with each other
assert set(test_dataset.filenames).isdisjoint(set(train_dataset.filenames))
assert set(test_dataset.filenames).isdisjoint(set(valid_dataset.filenames))
assert set(train_dataset.filenames).isdisjoint(set(valid_dataset.filenames))
print(f"Train size: {len(train_dataset)}")
print(f"Valid size: {len(valid_dataset)}")
print(f"Test size: {len(test_dataset)}")
n_cpu = os.cpu_count()
train_dataloader = DataLoader(train_dataset, batch_size=16, shuffle=True, num_workers=n_cpu)
valid_dataloader = DataLoader(valid_dataset, batch_size=16, shuffle=False, num_workers=n_cpu)
test_dataloader = DataLoader(test_dataset, batch_size=16, shuffle=False, num_workers=n_cpu)
- 2~4行目:ダウンロードした、データセットから、学習、検証、テストデータをそれぞれ、セットします。(2行目が学習、3行目が検証、4行目がテストデータです)
- 7~9行目:データのエラーチェックをしておきます。ここで入れておくと原因がはっきりしていいですね」。
- 11~13行目:学習、検証、テストのデータ数を表示します
- 15行目:cpuの数
- 16~18行目:データローダーの定義です。
引数は
batchsizeが1回で読み込むデータの数16、
shuffleはシャフルするのか、順番通りに読み込むのか、
num_workersは使用するcpuの数
# lets look at some samples
sample = train_dataset[0]
plt.subplot(1,2,1)
plt.imshow(sample["image"].transpose(1, 2, 0)) # for visualization we have to transpose back to HWC
plt.subplot(1,2,2)
plt.imshow(sample["mask"].squeeze()) # for visualization we have to remove 3rd dimension of mask
plt.show()
sample = valid_dataset[0]
plt.subplot(1,2,1)
plt.imshow(sample["image"].transpose(1, 2, 0)) # for visualization we have to transpose back to HWC
plt.subplot(1,2,2)
plt.imshow(sample["mask"].squeeze()) # for visualization we have to remove 3rd dimension of mask
plt.show()
sample = test_dataset[0]
plt.subplot(1,2,1)
plt.imshow(sample["image"].transpose(1, 2, 0)) # for visualization we have to transpose back to HWC
plt.subplot(1,2,2)
plt.imshow(sample["mask"].squeeze()) # for visualization we have to remove 3rd dimension of mask
plt.show()
試しに、画像を見てみます
- 3行目:学習データセットの0番を変数sampleへ
- 4行目:1行2列の箱を作っておいて、1番目(左側)を指定。
- 5行目:データの”image”画像を、transposeで次元を入れ替えて(R,G,B)へ変換して。画像表示
- 6行目:データの”mask”画像(犬・猫にマスク)をsqueezeで1次元削ってから画像表示。3次元データを2次元データにして、マスク描画できるよにしています。
- 7行目:表示のおまじない
- 10~15行目は同様に、検証データ
- 17~22行目は同様に、テストデータ
モデル
一番大事なモデル関連のクラスを作成します。長いです。。
class PetModel(pl.LightningModule):
def __init__(self, arch, encoder_name, in_channels, out_classes, **kwargs):
super().__init__()
self.model = smp.create_model(
arch, encoder_name=encoder_name, in_channels=in_channels, classes=out_classes, **kwargs
)
# preprocessing parameteres for image
params = smp.encoders.get_preprocessing_params(encoder_name)
self.register_buffer("std", torch.tensor(params["std"]).view(1, 3, 1, 1))
self.register_buffer("mean", torch.tensor(params["mean"]).view(1, 3, 1, 1))
# for image segmentation dice loss could be the best first choice
self.loss_fn = smp.losses.DiceLoss(smp.losses.BINARY_MODE, from_logits=True)
def forward(self, image):
# normalize image here
image = (image - self.mean) / self.std
mask = self.model(image)
return mask
def shared_step(self, batch, stage):
image = batch["image"]
# Shape of the image should be (batch_size, num_channels, height, width)
# if you work with grayscale images, expand channels dim to have [batch_size, 1, height, width]
assert image.ndim == 4
# Check that image dimensions are divisible by 32,
# encoder and decoder connected by `skip connections` and usually encoder have 5 stages of
# downsampling by factor 2 (2 ^ 5 = 32); e.g. if we have image with shape 65x65 we will have
# following shapes of features in encoder and decoder: 84, 42, 21, 10, 5 -> 5, 10, 20, 40, 80
# and we will get an error trying to concat these features
h, w = image.shape[2:]
assert h % 32 == 0 and w % 32 == 0
mask = batch["mask"]
# Shape of the mask should be [batch_size, num_classes, height, width]
# for binary segmentation num_classes = 1
assert mask.ndim == 4
# Check that mask values in between 0 and 1, NOT 0 and 255 for binary segmentation
assert mask.max() <= 1.0 and mask.min() >= 0
logits_mask = self.forward(image)
# Predicted mask contains logits, and loss_fn param `from_logits` is set to True
loss = self.loss_fn(logits_mask, mask)
# Lets compute metrics for some threshold
# first convert mask values to probabilities, then
# apply thresholding
prob_mask = logits_mask.sigmoid()
pred_mask = (prob_mask > 0.5).float()
# We will compute IoU metric by two ways
# 1. dataset-wise
# 2. image-wise
# but for now we just compute true positive, false positive, false negative and
# true negative 'pixels' for each image and class
# these values will be aggregated in the end of an epoch
tp, fp, fn, tn = smp.metrics.get_stats(pred_mask.long(), mask.long(), mode="binary")
return {
"loss": loss,
"tp": tp,
"fp": fp,
"fn": fn,
"tn": tn,
}
def shared_epoch_end(self, outputs, stage):
# aggregate step metics
tp = torch.cat([x["tp"] for x in outputs])
fp = torch.cat([x["fp"] for x in outputs])
fn = torch.cat([x["fn"] for x in outputs])
tn = torch.cat([x["tn"] for x in outputs])
# per image IoU means that we first calculate IoU score for each image
# and then compute mean over these scores
per_image_iou = smp.metrics.iou_score(tp, fp, fn, tn, reduction="micro-imagewise")
# dataset IoU means that we aggregate intersection and union over whole dataset
# and then compute IoU score. The difference between dataset_iou and per_image_iou scores
# in this particular case will not be much, however for dataset
# with "empty" images (images without target class) a large gap could be observed.
# Empty images influence a lot on per_image_iou and much less on dataset_iou.
dataset_iou = smp.metrics.iou_score(tp, fp, fn, tn, reduction="micro")
metrics = {
f"{stage}_per_image_iou": per_image_iou,
f"{stage}_dataset_iou": dataset_iou,
}
self.log_dict(metrics, prog_bar=True)
def training_step(self, batch, batch_idx):
return self.shared_step(batch, "train")
def training_epoch_end(self, outputs):
return self.shared_epoch_end(outputs, "train")
def validation_step(self, batch, batch_idx):
return self.shared_step(batch, "valid")
def validation_epoch_end(self, outputs):
return self.shared_epoch_end(outputs, "valid")
def test_step(self, batch, batch_idx):
return self.shared_step(batch, "test")
def test_epoch_end(self, outputs):
return self.shared_epoch_end(outputs, "test")
def configure_optimizers(self):
return torch.optim.Adam(self.parameters(), lr=0.0001)
- 1行目:pl.LightningModuleを継承してクラスを作成します。名前はPetModelです。
- 3~15行目:初期設定の関数を定義していきます。
- 5~7行目:”Segmentation models”のメソッド、smp.create_modelを使ってモデルを作成します。引数は
archがアルゴリズムの名前、encder_nameがエンコーダ(圧縮側モデル)の名前、in_channelsが入力画像のチャンネル数(RGBなら3)、classesが出力のクラスの数(今回は、1クラスですね)、 - 10~12行目:画像前処理用の数値を算出しています
- 10行目:前処理用の数値を取得します。
- 11行目:画像の輝度の標準偏差を求めています。viewで次元を(1,3,1,1)に変更しています。
- 12行目:画像の輝度の平均値を求めています。同じくviewで次元を変更しています。
- 15行目:損失関数の定義です。DiceLossを推奨しています。2値分類なのでBinarymodeにしているようです。
- 17~21行目:順伝搬の関数定義です
- 19行目:画像を標準化します。(平均値で引いて、標準偏差で割る)
- 20行目:モデルに画像を入力して、mask画像(セグメンテーション結果)を出力します。
- 23行目:モデルの。。。。。
- 25行目:Batchから画像を取り出して
- 29行目:画像の次元が4次元かチェックします。(バッチサイズ、チャンネル数、高さ、幅 の4次元が正解です)
- 36行目:画像の幅と高さを取り出して
- 37行目:幅と高さが32で割り切れるか(32の倍数か)チェックします。32の倍数でないとモデルが上手く回りません。
- 39行目:Batchから”mask”を読み込んで
- 43行目:maskの次元が4かチェックします。
- 46行目:maskの最大値が1以下で、最小値が0以上かチェックします
- 48行目:順伝搬させて、logitsを出力します
- 51行目:モデル出力と、正解maskを比較して、lossを計算します
- 56行目:logits_maskにsigmoid関数をかけて、確率に変換します(犬・猫である確率ですね)
- 58行目:確率を閾値0.5で、判定します。(0.5より大きければ、犬・猫、0.5以下ならば背景)
- 65行目:精度を計算します。
tpが true positive(予測結果が犬・猫で正解だったpixel)、fpがfalse positive(予測結果が背景で不正解だったpixel)、fnがfalse negative(予測結果が背景で不正解だったpixel)、tnがtrue negative(予測結果が背景で正解だったpixel) です。 - 75行目:最後のepochで行う精度計算です
- 77~80行目:tp,fp,fn,tnを全部の画像分結合します。
- 84行目:tp,fp,fn,tnからIoUという精度を計算します。ここでは各画像ごとのIoUが出力されます。IoUについては、他の皆さんの説明ページを参考になさってください。
- 91行目:データセット全体のIoUを計算します。
- 93~96行目:IoUを辞書形式でmetricsという変数に保存しておきます。
- 98行目:log_dictに、metricsを保存しておきます。
- 100~101行目:学習ステップの処理関数
- 103~104行目:学習の最後のエポックでの処理関数
- 106~107行目:バリデーションステップの処理関数
- 109~110行目:バリデーションの最後のエポックの処理関数
- 112~113行目:テストの処理関数
- 115~114行目:テストの最後のエポックでの処理関数
- 118~119行目:オプティマイザの定義。Adamですね。学習率のデフォルトは、lr=0.0001
model = PetModel("FPN", "resnet34", in_channels=3, out_classes=1)
上で頑張って作ったクラスを使って、モデルを作成します。
”FPN”は、 fully convolution neural network (全部畳み込みのニューラルネットワーク)です。 encoderは”resnet34″ですね。
学習
trainer = pl.Trainer(
gpus=1,
max_epochs=5,
)
trainer.fit(
model,
train_dataloaders=train_dataloader,
val_dataloaders=valid_dataloader,
)
実際の学習部分は簡単ですね。
- 1~4行目:学習の設定、gpusでgpuの番号指定、max_epochsでエポック数を設定します。
- 6~10行目:学習実行、モデルと、学習用データローダ、バリデーション用データローダを引数で指定します。
バリデーションとテストの 精度
# run validation dataset
valid_metrics = trainer.validate(model, dataloaders=valid_dataloader, verbose=False)
pprint(valid_metrics)
- 2行目:バリデーションデータに対する精度を計算します。引数でモデルと、データローダを指定します
# run test dataset
test_metrics = trainer.test(model, dataloaders=test_dataloader, verbose=False)
pprint(test_metrics)
同じくテストデータの精度も計算します。
結果の可視化
batch = next(iter(test_dataloader))
with torch.no_grad():
model.eval()
logits = model(batch["image"])
pr_masks = logits.sigmoid()
for image, gt_mask, pr_mask in zip(batch["image"], batch["mask"], pr_masks):
plt.figure(figsize=(10, 5))
plt.subplot(1, 3, 1)
plt.imshow(image.numpy().transpose(1, 2, 0)) # convert CHW -> HWC
plt.title("Image")
plt.axis("off")
plt.subplot(1, 3, 2)
plt.imshow(gt_mask.numpy().squeeze()) # just squeeze classes dim, because we have only one class
plt.title("Ground truth")
plt.axis("off")
plt.subplot(1, 3, 3)
plt.imshow(pr_mask.numpy().squeeze()) # just squeeze classes dim, because we have only one class
plt.title("Prediction")
plt.axis("off")
plt.show()
推論結果を可視化して、確認します。
- 1行目:テストデータセットから、1バッチ分取り出します。
- 2行目:重みを更新しないモードで
- 3行目:モデルを評価モードに
- 4行目:モデルに画像を入力して、logitsを計算
- 5行目:logitsにsigmoid関数をかけてmask画像を作成
- 7行目:画像データ、マスク画像(正解)、マスク画像(推論)を順番に読み込みます
- 8~13行目:画像の表示です。
- 8行目:figureのサイズ設定
- 10行目:1行3列の中の一番左に表示
- 11行目:画像を表示。transposeで次元の配列を変更しています。(チャンネル、高さ、幅)→(高さ、幅、チャンネル)
- 12行目:タイトルは”Image”
- 13行目:グラフの軸は邪魔なので表示しない
- 15~18行目:同じように正解マスク画像の表示です
- 20~23行目:同じように推論したマスク画像の表示です。
まとめ
“Segmentation models”を使った、セグメンテーションのサンプルコードを解説しました。
コンペ参加者も利用しており、比較的に簡単に学習、推論が実装できますので、ぜひ身に着けてください。